Robust-M new two-parameter estimator for linear regression models: Simulations and applications
Keywords:
Ordinary least squares, Multicollinearity, Outliers, Estimators, Simulation studyAbstract
In the presence of multicollinearity and outliers, the ordinary least squares estimator remains inconsistent and unreliable. Several estimators have been proposed that can co-handle the problems of multicollinearity and outliers simultaneously. However, there is still a need to explore some other robust methods when the two anomalies appear in the linear regression model and recommend it to end users of statistics. Therefore, this study proposed Robust-M New Two Parameter (RNTP) and examined its performance over some already existing ones in the presence of multicollinearity and outliers in the x-direction. The theoretical expression under some conditions was established to showcase the new estimator's superiority. A simulation study was carried out alongside some factors to show that the RNTP is better than all other estimators considered in the study. The simulation study results revealed that RNTP outperformed other estimators in the study using the minimum MSE as the criterion. Likewise, real-life data was applied to affirm this claim.
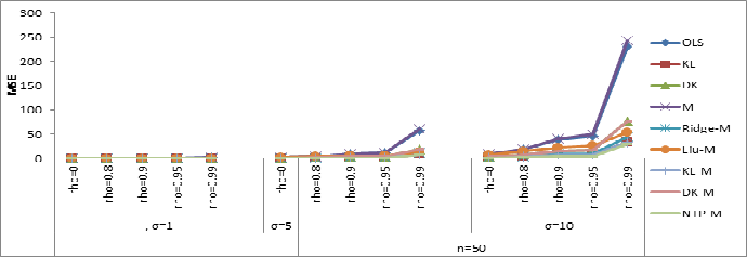
Published
How to Cite
Issue
Section
Copyright (c) 2023 Taiwo J. Adejumo, Kayode Ayinde, Abayomi A. Akomolafe, Olusola S. Makinde, Adegoke S. Ajiboye

This work is licensed under a Creative Commons Attribution 4.0 International License.