Robust weighted ridge regression based on S – estimator
Keywords:
Outliers, Weighted Ridge, Multicollinearity, S Estimator, HeteroscedasticityAbstract
Ordinary least squares (OLS) estimator performance is seriously threatened by correlated regressors often called multicollinearity. Multicollinearity is a situation when there is strong relationship between any two exogenous variables. In this case, the ridge estimator offers more reliable estimations when such scenario occurs. Outliers can have significant impact on the estimation of regression parameters leading to biased results. However, both OLS and Ridge estimators are sensitive to outlaying observations (outliers). The outlier – prone dataset in the endogenous variable has been efficiently solved utilizing the Robust M estimator in our recent paper. In recent studies, Robust M estimator has some drawbacks despite its efficient performances with outlier – prone dataset in the dependent (endogenous) variable hence the consideration of the Robust S estimator. The Robust S estimator is less sensitive to outliers in both endogenous and exogenous variables and utilizes standard deviation rather than the median in the case of Robust M estimator to handle outliers in the endogenous variable. The Robust ridge based on the S estimator was well suited to model dataset with multicollinearity and outliers problems in the endogenous variable. Also, it was noted in literature that outliers are one of the causes of heteroscedasticity and non – robust weighted least squares were initially employed to figure out for them. In this study, we introduced proposed Robust S weighted ridge estimator by adding a novel weighting method to address the three problems in a linear regression model. The selected Ridge estimators and Robust S estimator in two weighted versions (True weight (Wo) and suggested new weight (W1)) were combined to respectively develop the Robust S Ridge and Robust S Weighted Ridge estimators. Monte – Carlo simulation experiments were conducted on a linear regression model with three and six exogenous variables exhibiting different degrees of Multicollinearity, with heteroscedasticity structure of powers, size of outlier in endogenous variable and error variances and five levels of sample sizes. The Mean Square Error (MSE) was used as a criterion to evaluate the performances of the new and existing estimators. Simulation findings show categorically that the new approach is preferred over previous approach and hereby recommended.
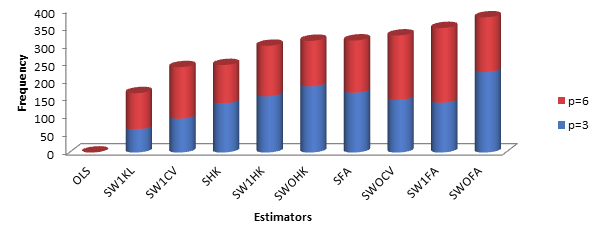
Published
How to Cite
Issue
Section
Copyright (c) 2023 Taiwo Stephen Fayose, Kayode Ayinde, Olatayo Olusegun Alabi, Abimbola Hamidu Bello

This work is licensed under a Creative Commons Attribution 4.0 International License.
How to Cite
Similar Articles
- Taiwo Stephen Fayose, Kayode Ayinde, Olatayo Olusegun Alabi, M Robust Weighted Ridge Estimator in Linear Regression Model , African Scientific Reports: Volume 2, Issue 2, August 2023
- Abiola T. Owolabi, Kayode Ayinde, Olusegun O. Alabi, A Modified Two Parameter Estimator with Different Forms of Biasing Parameters in the Linear Regression Model , African Scientific Reports: Volume 1, Issue 3, December 2022
- Janet Iyabo Idowu, Olasunkanmi James Oladapo, Abiola Timothy Owolabi, Kayode Ayinde, On the biased Two-Parameter Estimator to Combat Multicollinearity in Linear Regression Model , African Scientific Reports: Volume 1, Issue 3, December 2022
- Taiwo J. Adejumo, Kayode Ayinde, Abayomi A. Akomolafe, Olusola S. Makinde, Adegoke S. Ajiboye, Robust-M new two-parameter estimator for linear regression models: Simulations and applications , African Scientific Reports: Volume 2, Issue 3, December 2023
- E. S. Okonofua, N. Kayode-Ojo, Urban Flooding Vulnerability Analysis Using Weighted Linear Method With Geospatial Information System , African Scientific Reports: Volume 2, Issue 3, December 2023
You may also start an advanced similarity search for this article.
Most read articles by the same author(s)
- Abiola T. Owolabi, Kayode Ayinde, Olusegun O. Alabi, A Modified Two Parameter Estimator with Different Forms of Biasing Parameters in the Linear Regression Model , African Scientific Reports: Volume 1, Issue 3, December 2022
- Janet Iyabo Idowu, Olasunkanmi James Oladapo, Abiola Timothy Owolabi, Kayode Ayinde, On the biased Two-Parameter Estimator to Combat Multicollinearity in Linear Regression Model , African Scientific Reports: Volume 1, Issue 3, December 2022
- Taiwo Stephen Fayose, Kayode Ayinde, Olatayo Olusegun Alabi, M Robust Weighted Ridge Estimator in Linear Regression Model , African Scientific Reports: Volume 2, Issue 2, August 2023