A Modified Two Parameter Estimator with Different Forms of Biasing Parameters in the Linear Regression Model
Keywords:
Ridge estimator, Liu estimator, Multicollinearity, Mean square error, Kibria-Lukman estimator, Prior iInformationAbstract
Despite its common usage in estimating the linear regression model parameters, the ordinary least squares estimator often suffers a breakdown when two or more predictor variables are strongly correlated. This study proposes an alternative estimator to the OLS and other existing ridge-type estimators to tackle the problem of correlated regressors (multicollinearity). The properties of the proposed estimator were derived, and six forms of biasing parameter k (generalized, median, mid-range, arithmetic, harmonic and geometric means) were used in the proposed estimator to compare its performance with five other existing estimators through a simulation study. The proposed estimator dominated existing estimators when the mid-range, arithmetic mean, and median versions of k were used. However, the proposed estimator did not perform well when the generalized, harmonic, and geometric
mean versions were used.
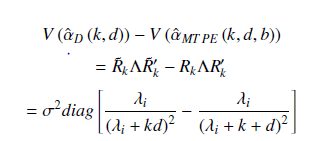
Published
How to Cite
Issue
Section
Copyright (c) 2022 Abiola T. Owolabi, Kayode Ayinde, Olusegun O. Alabi

This work is licensed under a Creative Commons Attribution 4.0 International License.