Investigating factors influencing PM2.5 threshold exceedance in Nigeria using multivariate logistic regression analysis
Keywords:
PM2.5 threshold exceedance, Logistic Regression Model, Meteorological Variables , Aerosol Emission Rate Variables, NigeriaAbstract
The understanding of the drivers of fine particulate matter of diameter less than or equal to 2.5 micrometers (PM2.5) in Nigeria will greatly assist policymakers and other stakeholders in developing appropriate air quality management strategies to protect the health of the public. This research analyzed the drivers of PM2.5 threshold exceedance (PTE) in Nigeria from 2000 to 2022, employing a multivariate logistic regression (MLR) model. The work assessed aerosol emission variables (AEVs) and meteorology variables (MEVs) to understand their impact on PM2.5 concentrations. Results showed a significant spatial heterogeneity in the trend of PM2.5 concentrations, with increasing values in all the study areas. The estimated coefficients of black carbon emission (BCEM) and sulfate emission (SUEM) showed a significant positive response, exacerbating the threshold exceedance in Abuja and Lagos, while dust emission (DUEM) had a significant positive response in Kano and Lagos. On the other hand, OCEM had a negative association in all the study locations. The MEV variables of sea level pressure (SLP), relative humidity (RH), planetary boundary layer height (PBLH), and wind speed (WS) had significant negative responses in Abuja, while RH and WS were significant in Kano, and RH, PBLH, and precipitation (P) were significant in Lagos, indicating that the PM2.5 concentrations decrease with increasing values of these factors; however, temperature (TMP) had a significant positive response in Lagos but was not significant in Abuja and Kano. The output of the study revealed complex relationships between aerosol emissions, climate, and other environmental variables driving the PTE in Nigeria.
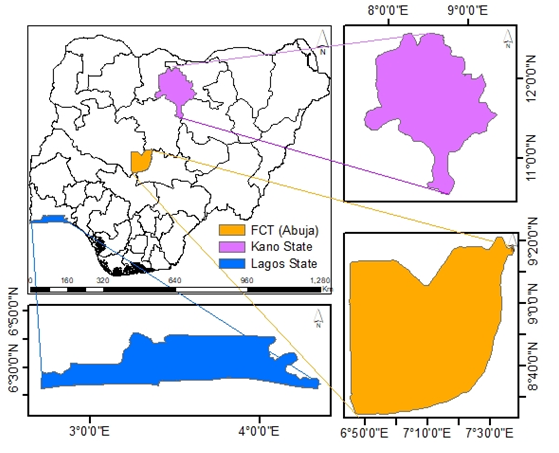
Published
How to Cite
Issue
Section
Copyright (c) 2024 Tertsea Igbawua, Aondongu Alexander Tyovenda, Terver Sombo, Idugba Mathias Echi, Fanan Ujoh

This work is licensed under a Creative Commons Attribution 4.0 International License.