Enhanced machine learning model for classification of the impact of technostress in the COVID and post-COVID era
Keywords:
COVID-19 era, Technostress, Machine Learning Models, Machine Learning, AI, Deep LearningAbstract
The global crisis caused by the coronavirus outbreak and other diseases has significantly changed daily life, work, and education, forcing individuals and organizations to adapt to evolving virtual environments. These challenges have led to conditions induced by an inability to process information effectively with computer technologies. This study models a system that employs a Random Forest algorithm for prediction and classification, using age, gender, hours spent, and technological experience as parameters to categorize stress into high, moderate, and low levels. Data were collected via a questionnaire during the COVID-19 and post-COVID eras using a non-probabilistic sample of knowledgeable respondents. The model achieved 90% accuracy, demonstrating its prediction efficiency. Additionally, an interactive user interface was developed to facilitate real-time evaluation of technostress’s impact on technology use. This work contributes a novel machine learning framework for technostress assessment, providing a practical tool for organizations and policymakers to better understand and mitigate technology-induced stress.
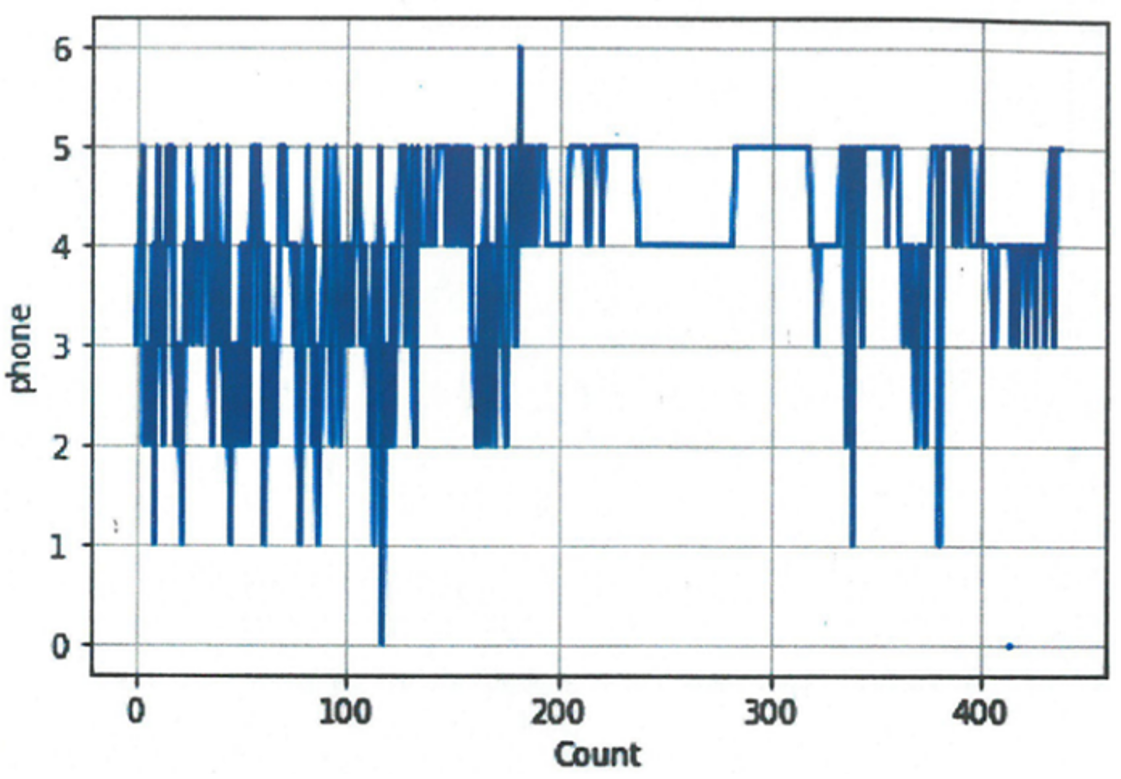
Published
How to Cite
Issue
Section
Copyright (c) 2025 Gabriel James, Anietie Ekong, Aloysius Akpanobong, Enefiok Etuk, Saviour Inyang, Samuel Oyong, Ifeoma Ohaeri, Chikodili Orazulume, Peace Okafor

This work is licensed under a Creative Commons Attribution 4.0 International License.